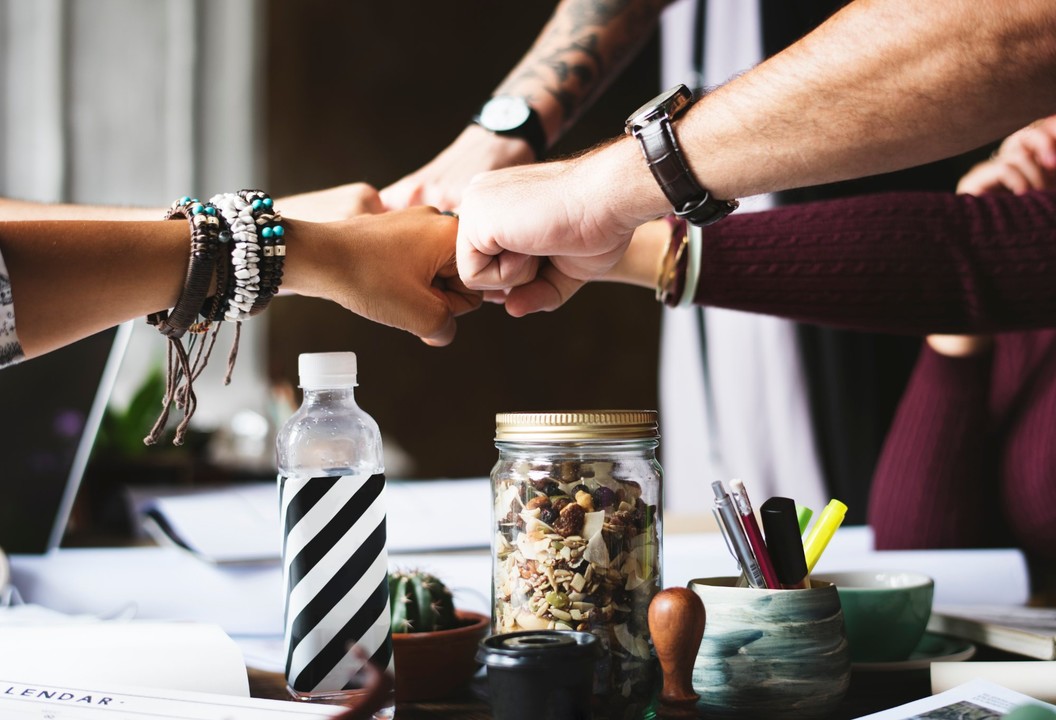
The global labor market is tight and highly competitive today. Enterprises – private, nonprofit, educational and civic – are all dealing with unemployment rates that are historically low and voluntary resignations that are at an all-time high. Not surprisingly then, managing employee turnover is now an HR metric of highest priority. Why? Because when employees quit they not only leave an organization shorthanded but also take the substantial value of them.
Employee turnover is extremely costly for an organization. The consequences of turnover are magnified for organizations with occupations that require specific education or training. Rehiring and retraining that caliber of lost talent will likely be more time-consuming and very expensive. According to a SHRM (Society for Human Resource Management) study, direct and indirect employee replacement costs can range from 30% to 150% of an employee’s annual salary, depending on the level of employment from entry/non-skilled through a supervisor. The replacement cost for senior executives can climb to over 200%.
Fortunately, organizations have more control over attrition than they think. They just need to know how to read the signs. Technology today provides powerful techniques for both predicting and mitigating individual employee turnover.
The Era of Workforce Analytics
Gaining insight – the capacity to obtain an accurate and deep intuitive understanding – is the primary benefit of predictive analytics. In the case of workforce turnover, predictive analytics can both help you understand why an employee quit as well as tell you to how to prevent losing one.
Know that employee attrition can be controlled. Predictive analytics starts by identifying a set of parameters that influence the employees and the organization. There will be parameters that are foreseeable, such as retirement age, childbirth or planned medical treatment. Alternatively, some are unforeseeable, such as funding or operating performance, office relocation or management change. In all these instances, analytical models developed as a result of data analysis can identify who is going to leave, when and why.
Setting parameters
Predictive algorithms, such as those utilized by QuitAlert, enable an organization to obtain a clear understanding of, as well as an advanced capability to undertake preventive measures for, employee attrition. Essentially, the process starts by clustering and classifying employee profiles based on multiple attributes, such as education level, work experience, distance from hometown, age, gender, marital status, etc. These data points will generate multiple ‘risk of attrition’ layers. The database can be expanded to include more parameters like performance over the years, pay raise frequency, advanced skills and training, etc.
The accuracy and value of the predictive model being developed will be directly affected by the quality and range of the parameters selected. In other words, parameters guide the determination of which ‘type’ of a predictive model is best suited to meet the needs of the organization.
Here is one of the example that shows the approach of QuitAlert to predict the employees who re going to quit within 3 to 6 months;
Interpreting Behavioral Analysis Plot:
The red isohypses illustrate the varying intensity of zones of attitudes shared by both your active and passive employees. These zones represent the regions of greatest concern. The green points (active employees) displayed in close proximity to red isohypses, or within intensive red isohypse zones, are more likely to behave like the red points (passive employees) who previously left. These green points are then identified as a high flight risk or likely to move employees.
Determining which predictive model to use
Analytics extends beyond measuring and describing the past to predicting what is likely to happen and optimizing what should happen. There are three types of analytics:
- Descriptive: Analytics that help you understand how things are going.
- Predictive: Analytics that help you forecast future performance and results.
- Prescriptive: Analytics that suggest a prescribed step or action.
Artificial intelligence and machine learning algorithms are now available that will construct the predictive model that bests serves an organization’s particular needs. Among the options is the ‘classification’ model that catalogs the employees based on their risk to leave the company. There is also the ‘non-linear regression’ model, which gives the ‘probability of attrition’ when the outcomes are in direct opposition.
Should employee turnover need to be based on additional factors such as a Gini index and other ratios, use of a ‘decision tree’ model may be appropriate. Understand that the decision tree model can become very large and complex when it draws upon multiple parameters.
The ‘random forest’ method, which combines several decision trees using multiple algorithms to classify and understand complexities and predictions, may be the best approach when an especially broad analysis is required.
While each of these models is designed to provide accurate predictability, seamless implementation will rely on selecting the right model. The decision on which model to engage will be based on the parameters, data availability, budget, computational power and the requirements of decision makers.